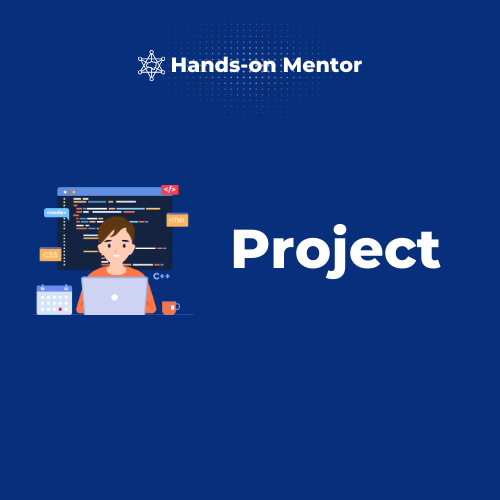
Project Description
Retail store performance analysis is critical for evaluating stores' sales, customer satisfaction and operational efficiency. In this project, we will evaluate store performance, customer behavior and inventory management by analyzing sales data, customer data, store information and inventory data. Our goal is to help store managers and retail analysts improve store performance and make strategic decisions.
Project Usage Areas
This project has several uses for retail store managers, retail analysts and data scientists:
Sales Performance Analysis: Improving performance and identifying sales trends by analyzing sales data.
Customer Behavior: Evaluating customer behavior and loyalty by analyzing customer data.
Inventory Management: Managing stock levels and reorder needs by analyzing inventory data.
Store Efficiency: Increasing operational efficiency by analyzing store information.
Strategic Decisions: Developing and improving store management strategies using data analysis.
Dataset Description
The data set to be used in this project includes the data needed to evaluate retail store performance. The dataset consists of four main files in total:
Sales Data (sales_data)
SalesID: Sales ID
StoreID: Store ID
ProductID: Product ID
CustomerID: Customer ID
SalesDate: Sales date
SalesAmount: Sales amount
Quantity: Quantity sold
Customer Data (customer_data)
CustomerID: Customer ID
CustomerName: Customer name
Age: Age Gender: Sex
city: city
LoyaltyScore: Loyalty score
Store Information (store_data)
StoreID: Store ID
StoreName: Store name
Location: Location
SquareFeet: Store area (ft²)
Manager: Store manager
Inventory Data (inventory_data)
InventoryID: Inventory ID
StoreID: Store ID
ProductID: Product ID
StockLevel: Stock level
ReorderLevel: Reorder level
There are various dirty data problems in this dataset, such as missing data, outlier data, and wrong data type. This is an ideal data set to experience data cleaning and processing processes commonly encountered in real life.
Student Benefits
This project provides many benefits for students:
Data Manipulation: Students develop skills in examining, cleaning, and analyzing data sets.
Using Pandas: They learn to use the data processing and analysis methods of the Pandas library effectively.
Data Cleaning: They gain skills in cleaning missing data, outliers and incorrect data types.
Business Intelligence: By analyzing data sets, they improve their ability to evaluate store performance and customer behavior and make strategic decisions.
Reporting: Provides skills to effectively report and present analysis results.
Real Life Applications: Provides practical information about data problems and analysis processes encountered in real life.
If you want to lay the foundations of Python and gain competence in data analysis and science, you can immediately register for a 1-month intensive Python camp. Take a look now at the interactive and practice-oriented training program developed in Helsinki, inspired by Finnish education models, consisting of ~40 hours of live lessons, ~50 comprehensive projects, ~15 quizzes and countless coding exercises!